AI insights for smarter lead time management.
About the Company
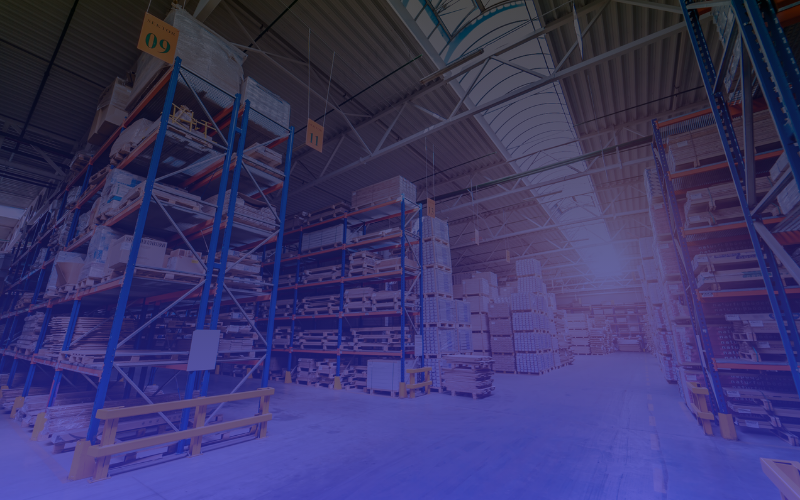
Annual Revenue: $800M (2022)
Locations: 100 stores worldwide
Innovation Focus: Committed to adopting cutting-edge technology to stay ahead in a competitive market.
Employees: 3,800
The client is a retailer offering a diverse range of products across 100 local locations. Despite its growth, the company faced significant operational challenges due to inefficient supply chain management.
Challenge
The company encountered significant difficulties in predicting inbound lead times using a 12-week rolling average method, which failed to consider specific factors such as SKU, supplier, and carrier. This oversight resulted in inventory congestion, transportation penalties, and elevated labor expenses.
The company faced several ongoing challenges that hindered its ability to forecast order arrivals and generate real-time predictions:
- Statistical anomalies were hard to identify due to inadequate or missing timestamps, and the poor quality of carrier data complicated the process of masking these issues for AI algorithm training.
- Predicting delays was challenging without access to Automatic Identification Systems (AIS) data, which is essential for developing port congestion features in AI models.
- Data scientists lacked the ability to monitor or segment models producing rolling average lead times, compounded by the absence of accurate AIS shipment network data.
- The retailer struggled with operational tasks and predictive capabilities due to insufficient staffing, including a shortage of data scientists and data engineers, preventing effective data handling and analysis.
Consequently, these challenges led to the small retailer experiencing stockpiles at ports and distribution centers, incurring transportation fines for emergency shipments, and incurring higher labor costs from unnecessary over-staffing.
Our Approach
OneGen AI has introduced an innovative AI-driven Supply Chain Analysis solution, structured around two principal machine learning components:
- Static Prediction Model: This model generates a one-time forecast for the expected delivery date at the moment a purchase order (PO) is created.
- Dynamic Tracking Model: Continuously updates the estimated time of arrival (ETA) for containers from the moment they leave a port until they arrive at their destination.
Deployment and Performance:
The system was implemented in three key regional port areas, collectively responsible for managing about 50% of the total import volume. Extensive validation of the models was conducted using datasets from both pre-COVID and more recent periods, confirming consistent effectiveness.
User Interface and Model Management:
- Visual Interface: A comprehensive multi-screen interface was designed to display AI insights and track order delays, enabling users to visually and temporally follow the order’s journey and pinpoint locations responsible for delays.
- Model Operations (Model Ops): The data science team has access to advanced tools for monitoring and managing the predictive models, ensuring their continued accuracy and efficiency.
This robust setup enhances operational transparency and aids in the proactive management of supply chain risks.
Results
$10M in potential annual economic benefit for imported orders
$30M in potential annual economic benefit when scaled to domestically-sourced orders
65% improvement in lead time predictions for estimated delivery dates
35% improvement in daily lead time predictions once orders were in transit
Solution Architecture
The OneGen AI platform leverages a comprehensive integration of diverse data sources and cutting-edge AI models to enhance supply chain management efficiency:
Data Sources Utilized:
- Internal Data: Includes purchase order history, inventory levels, sales data, and customer feedback.
- External Data: Comprises carrier schedules, port congestion information, and weather forecasts.
Platform Components:
- Data Integration Layer: Responsible for aggregating and cleansing data from all sources.
AI Models:
- Predictive Analytics: Uses historical and real-time data to forecast demand and lead times.
- Anomaly Detection: Scans for and identifies potential disruptions in real-time.
User Interface: Features dashboards for real-time monitoring and decision-making support.
Operational Flow:
- Data Collection: Data is systematically gathered from both internal and external sources.
- Data Processing: Data is cleaned and aggregated to prepare for analysis.
- Model Training: AI models are trained using historical data to enhance accuracy.
- Prediction: The system generates real-time predictions and insights based on processed data.
- Visualization: Insights are presented through intuitive and user-friendly dashboards.
This structured approach not only optimizes operations but also provides strategic insights that drive proactive decision-making in supply chain management
This structured approach not only optimizes operations but also provides strategic insights that drive proactive decision-making in supply chain management.
Project Highlights
Unified data from 12 enterprise data tables and three external data sources.
Developed 200+ time series analysis models.
Configured the OneGen AI Supply Network Risk application for real-time monitoring.
Engineering leaders from
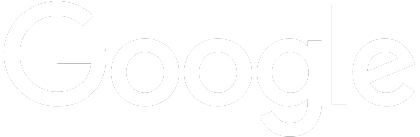
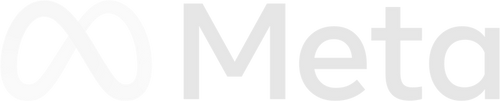
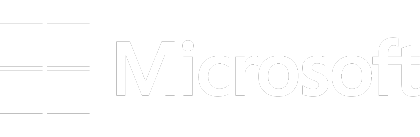
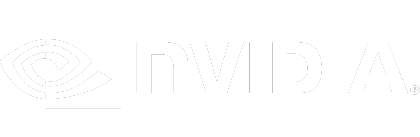
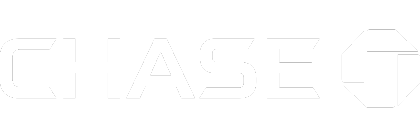
San Jose, CA - New York, NY