AI in healthcare: Use cases, applications, benefits, solution, AI agents and implementation
Detailed use cases, applications, benefits, solutions, AI agents, and implementation in Healthcare
The healthcare industry is one of the most complex and multifaceted sectors, facing challenges such as patient care, medical research, administrative efficiency, and regulatory compliance. These challenges are exacerbated by the need to manage massive healthcare data sets, including electronic health records (EHR), diagnostic imaging, genomic data, and real-time patient monitoring. As healthcare data continues to grow exponentially, traditional methods often struggle to keep pace with the demand for precision medicine and personalized care.
Artificial Intelligence (AI) in healthcare is revolutionizing the industry by offering advanced healthcare solutions that significantly improve the quality, efficiency, and accessibility of medical services. With AI technologies and machine learning (ML) algorithms, healthcare providers can analyze large datasets quickly and accurately, identifying trends, patterns, and insights that are otherwise hidden. This capability is crucial in medical diagnostics, where AI can assist healthcare professionals in detecting diseases earlier and with greater accuracy. According to Precedence Research, the global AI healthcare market reached an estimated value of USD 15.1 billion in 2022, with projections indicating growth to USD 187.95 billion by 2030, driven by a compound annual growth rate (CAGR) of 37% between 2022 and 2030. North America led the market with a notable valuation of USD 6.8 billion in 2022, reflecting the region’s adoption of AI in healthcare.
AI healthcare applications leverage data from multiple sources, such as EHR systems, diagnostic images, medical tests, and wearable health devices, to enhance clinical decision-making and treatment outcomes. In particular, AI’s ability to interpret medical imaging, including MRIs and CT scans, has led to earlier and more precise detection of critical illnesses like cancer and cardiovascular diseases. Moreover, AI in healthcare is transforming the management of chronic diseases such as diabetes, asthma, and heart failure by providing real-time monitoring, personalized treatment plans, and timely interventions. By integrating AI-powered healthcare solutions, providers can offer data-driven insights that enhance patient care and improve overall healthcare outcomes.
AI-driven tools are also reshaping other critical areas of healthcare. For instance, AI for medical imaging improves diagnostic accuracy, AI in drug discovery accelerates the analysis of complex biological data, and AI-powered virtual assistants enhance patient engagement by providing personalized health recommendations and support. The integration of AI in healthcare not only reduces administrative burdens but also elevates patient-centered care, making healthcare delivery more accurate, efficient, and accessible to all.
This article will dive into AI’s role in healthcare, discussing key applications like AI for diagnostics, AI for personalized medicine, and AI-driven drug discovery. We will also explore the latest healthcare AI technologies and trends, as well as the benefits of adopting AI healthcare solutions to drive better patient outcomes, reduce costs, and optimize healthcare operations.
By incorporating AI into healthcare, providers can stay at the forefront of medical innovation, leveraging AI technology to transform patient care, enhance diagnostic accuracy, and improve healthcare delivery.
What is AI in healthcare? How artificial intelligence is transforming medical practices.
Artificial Intelligence (AI) in healthcare refers to the application of AI technologies to improve various aspects of the healthcare industry. This includes leveraging machine learning (ML), deep learning, natural language processing (NLP), and advanced data analysis techniques to process and interpret medical data. AI in healthcare enhances clinical decision-making, assists in medical diagnoses, automates administrative workflows, predicts disease outbreaks, and improves overall patient care. By analyzing large volumes of patient data, medical records, imaging results, and genetic profiles, AI enables healthcare professionals to extract valuable insights that lead to better clinical outcomes and greater operational efficiency.
A key advantage of AI in healthcare is its ability to accelerate diagnoses and improve patient outcomes through sophisticated data analysis. AI systems can identify patterns in medical imaging, patient histories, and real-time monitoring data, allowing for earlier detection of diseases and more accurate diagnostic results. A notable example is the work of PathAI, a company focused on improving pathology diagnoses. PathAI uses machine learning algorithms to analyze pathology slides with greater accuracy than traditional methods. Their AI technology assists pathologists in identifying diseases like cancer at earlier stages, ensuring more precise diagnoses and potentially life-saving interventions. By analyzing thousands of pathology images, the AI system helps reduce human error, improving both diagnostic accuracy and speed.
AI in healthcare also plays a crucial role in optimizing operational efficiency. AI-powered healthcare platforms can automate repetitive tasks such as medical data entry, appointment scheduling, and insurance claim processing, freeing up valuable time for healthcare staff to focus on patient care. In addition, AI’s predictive capabilities allow healthcare providers to foresee potential health trends, from hospital readmission risks to disease outbreaks, and take preventive actions accordingly.
As AI continues to evolve, its potential applications in healthcare are expanding rapidly. From improving diagnostic accuracy to streamlining administrative processes, AI offers significant opportunities to enhance patient outcomes, reduce healthcare costs, and increase the efficiency of healthcare systems. Moreover, AI-powered tools are being integrated into wearable devices and mobile apps, enabling patients to monitor their health in real time and share that data with their healthcare providers for better-informed care decisions.
This article will explore AI’s role in revolutionizing healthcare, focusing on use cases such as AI in pathology, AI in predictive analytics, and AI-driven automation. It will also discuss the significant advantages of adopting AI technologies to improve clinical decision-making, enhance patient outcomes, and streamline healthcare operations.
How does AI in healthcare work? Understanding the mechanics of AI-powered healthcare solutions.
Integrating AI into healthcare involves leveraging advanced technologies to improve data analysis, generate valuable insights, and support clinical decision-making. This approach transforms traditional healthcare workflows by incorporating large language models (LLMs) alongside an institution’s unique knowledge base, enabling real-time, data-driven decisions that enhance patient care and treatment outcomes.
This AI-driven architecture relies on various components working together to streamline healthcare processes. Below is a step-by-step breakdown of how this system functions:
- Data Sources: The process begins with collecting data from multiple healthcare-related sources, such as:
- Patient records: Comprehensive information on patient demographics, medical history, treatment plans, and outcomes.
- Clinical data: Real-time and historical data from electronic health records (EHRs), lab results, medical imaging, and diagnostic tests.
- Regulatory information: Documentation from health authorities, including compliance reports, safety alerts, and healthcare guidelines.
- Research articles: Insights from clinical studies, medical journals, and research publications that inform best practices.
- Healthcare market data: Information on healthcare costs, drug pricing, and insurance claims.
Data Pipelines: Once data is gathered, it moves through data pipelines where it is ingested, cleaned, and structured for analysis. These pipelines ensure the data is ready for efficient processing.
Embedding Model: The cleaned data is then processed by an embedding model, which converts textual information into numerical vectors. These vectors are essential for AI models to interpret and analyze the data. Leading embedding models include those from OpenAI, Google, and Cohere.
Vector Database: The processed vectors are stored in a vector database, allowing for efficient querying and retrieval of data. Popular vector databases include Pinecone, Weaviate, and PGvector, which optimize data management and accessibility.
APIs and Plugins: Various APIs and plugins, such as Serp, Zapier, and Wolfram, connect the different system components and enable additional functionalities like retrieving extra data or performing specific operations.
Orchestration Layer: This layer manages the entire workflow, ensuring seamless integration between all components. Tools like OneCare orchestrate tasks such as prompt chaining, managing API interactions, retrieving relevant data from vector databases, and preserving memory across multiple LLM calls. This step is crucial for preparing and sending queries to the LLM for processing.
Query Execution: The user initiates the process by submitting a query to the healthcare application. Queries may involve patient conditions, treatment options, or cost-related inquiries.
LLM Processing: The orchestration layer retrieves relevant data from the vector database and sends it to the language model for processing. The LLM analyzes the data and generates insights based on the query.
Output: The language model delivers outputs that may include summaries of patient conditions, treatment recommendations, or cost analyses. These insights are tailored to inform healthcare providers and administrators.
Healthcare Application: The processed output is presented in a user-friendly format within the healthcare application. This application organizes the data and insights, making it easy for medical professionals or administrators to interpret and act on the information.
Feedback Loop: User feedback on the AI-generated insights is critical for improving the accuracy and relevance of future outputs. This feedback loop ensures continuous refinement of the AI models over time.
AI Agent: Integrating AI agents into the architecture addresses complex healthcare challenges by interacting with external systems and enhancing decision-making through advanced reasoning and memory techniques.
LLM Cache: To improve system response times, tools like Redis, SQLite, and GPTCache cache frequently accessed information, ensuring faster data retrieval and processing.
Logging/LLMOps: Monitoring tools such as Weights & Biases, MLflow, Helicone, and Prompt Layer log actions and track system performance. These tools ensure that the AI models continue to operate optimally and that performance improvements are ongoing.
Validation: A validation layer is incorporated to ensure the accuracy and reliability of LLM outputs. Tools like Guardrails, Rebuff, Guidance, and LMQL provide safeguards that verify the quality of the AI-generated results.
LLM APIs and Hosting: To execute healthcare tasks and host applications, LLM APIs and hosting platforms are critical. Depending on project needs, developers can choose from APIs provided by OpenAI, Anthropic, or open-source models, and host these on cloud platforms like AWS, GCP, Azure, or specialized clouds like Databricks, Mosaic, and Anyscale.
This comprehensive approach highlights the transformative potential of AI in healthcare. By integrating diverse data sources with cutting-edge AI technologies, healthcare providers can generate precise, actionable insights that improve patient care, streamline operations, and support better decision-making across the industry. Through automation and real-time data processing, AI enhances healthcare efficiency and elevates the quality of care delivered to patients.
Integrate AI in Healthcare with Experts
Discover how AI can reinvent healthcare. Keen to upgrade your healthcare solutions?
How does AI address challenges across the healthcare workflow, from scheduling to post-treatment? AI’s role in improving efficiency and outcomes
Artificial Intelligence (AI) holds significant potential to address various challenges faced by healthcare businesses at every stage of the healthcare operations workflow. Here’s an overview of common issues and how AI can offer solutions:
Patient Scheduling and Registration
Challenge: Traditional healthcare appointment scheduling is often manual, leading to double bookings, no-shows, and long waiting times for patients. These inefficiencies result in poor resource management and a subpar patient experience.
Solution: AI-driven scheduling systems use historical data to optimize appointment slots by analyzing patient arrival patterns, appointment durations, and healthcare provider availability. AI can predict no-shows and overbookings, ensuring better utilization of appointment slots, reducing wait times, and improving resource efficiency.
Check-in Process
Challenge: Manual patient check-in processes can lead to errors, such as incorrect patient details or delayed data entry, slowing the patient intake process and affecting medical record accuracy.
Solution: AI-enabled chatbots and automated check-in systems streamline the process by guiding patients through prompts to collect necessary information. These systems reduce errors by verifying data in real time and integrating with electronic health records (EHRs), making the check-in process faster and more accurate, thereby enhancing the patient experience.
Clinical Assessment
Challenge: Variability in clinical decision-making among healthcare providers can lead to inconsistencies in patient care and treatment outcomes.
Solution: AI-powered decision support systems analyze patient data, such as medical history, lab results, and symptoms, to provide evidence-based insights and recommendations. These systems help standardize clinical assessments, reducing variability and enabling more informed decisions, leading to improved care quality.
Medical Examination and Diagnosis
Challenge: Access to specialists is often limited, particularly for patients in remote or underserved areas, resulting in delayed diagnosis and treatment.
Solution: AI diagnostic tools and telemedicine platforms enable remote consultations and assist in analyzing medical images and test results. AI helps healthcare providers diagnose conditions more accurately and quickly by providing second opinions or highlighting potential issues, especially in areas with limited specialist access.
Treatment Planning
Challenge: Creating personalized and effective treatment plans involves managing numerous variables, increasing the risk of errors in the process.
Solution: AI algorithms analyze patient data, including genetic information, lifestyle factors, and medical history, alongside the latest research. This allows for the development of individualized treatment plans that are evidence-based and continuously updated based on patient progress, ensuring optimal care.
Treatment Administration
Challenge: Medication errors, such as incorrect dosages, and challenges with patient adherence to prescribed treatments, are major concerns in healthcare.
Solution: AI-powered robotic systems ensure accurate medication administration by delivering the correct dosages at the right times. AI can also send personalized reminders and educational materials to patients, improving adherence to treatment plans and reducing errors.
Monitoring and Follow-up
Challenge: Continuous patient monitoring, especially for those with chronic conditions, can be resource-intensive and difficult to sustain.
Solution: AI-enabled remote patient monitoring systems, combined with wearable technology, track real-time health metrics and use AI analytics to detect early signs of health issues. This proactive approach reduces the need for frequent in-person visits and enables timely interventions, optimizing healthcare resources.
Claims Processing
Challenge: Slow and error-prone insurance claim processing can result in denials, impacting the financial stability of healthcare providers.
Solution: AI analyzes claims data to identify patterns and predict potential issues that could lead to denials. By ensuring claims are accurate and complete before submission, AI reduces denial rates and expedites the processing, improving financial outcomes for providers.
Payment Processing
Challenge: Managing payments and account reconciliation can be time-consuming, leading to delays in revenue collection and financial discrepancies.
Solution: AI-powered chatbots and virtual assistants manage payment-related inquiries and provide immediate assistance. Predictive analytics can forecast revenue cycles and improve cash flow management, ensuring timely payment collection and reconciliation.
Medical Records Management
Challenge: Manual record-keeping is prone to errors and consumes significant time, impacting the availability and accuracy of patient data.
Solution: AI-driven EHR systems automate record-keeping, ensuring accurate, up-to-date patient information. These systems also facilitate efficient data retrieval and exchange, improving collaboration among healthcare providers and enhancing patient care.
Quality Assurance and Compliance
Challenge: Adapting to frequently updated healthcare regulations requires significant resources and time, making it difficult for organizations to stay compliant.
Solution: AI can monitor regulatory changes and automatically assess their impact on healthcare practices. AI-driven compliance tools notify healthcare providers of necessary updates and streamline the verification process, reducing the risk of penalties and improving quality control.
Discharge Planning
Challenge: Lack of post-discharge support can lead to complications and readmissions, increasing healthcare costs and negatively impacting patient outcomes.
Solution: AI-powered care coordination tools create personalized discharge plans, including follow-up appointments, medication schedules, and self-care instructions. These tools provide ongoing support through automated follow-ups, ensuring that patients have the resources for a successful recovery.
Patient Education
Challenge: Educating patients about their health conditions and treatment options is essential, but healthcare providers often have limited time and resources for effective communication.
Solution: AI-powered educational platforms deliver personalized health information tailored to each patient’s understanding and preferences. These platforms enhance patient education, improving treatment adherence and empowering patients to manage their health more effectively.
Follow-up and Aftercare
Challenge: Monitoring patient outcomes post-treatment is crucial for long-term health, but resource constraints make this difficult to sustain.
Solution: AI analytics track patient outcomes by analyzing data from follow-up visits, wearable devices, and other sources. Early detection of trends or issues allows for timely interventions, improving aftercare and long-term health outcomes.
AI applications across the healthcare operations workflow can address critical challenges by enhancing efficiency, reducing errors, supporting clinical decisions, and ultimately delivering better patient care. However, successful AI implementation in healthcare must consider ethical, legal, and privacy issues to ensure a responsible and effective transformation.
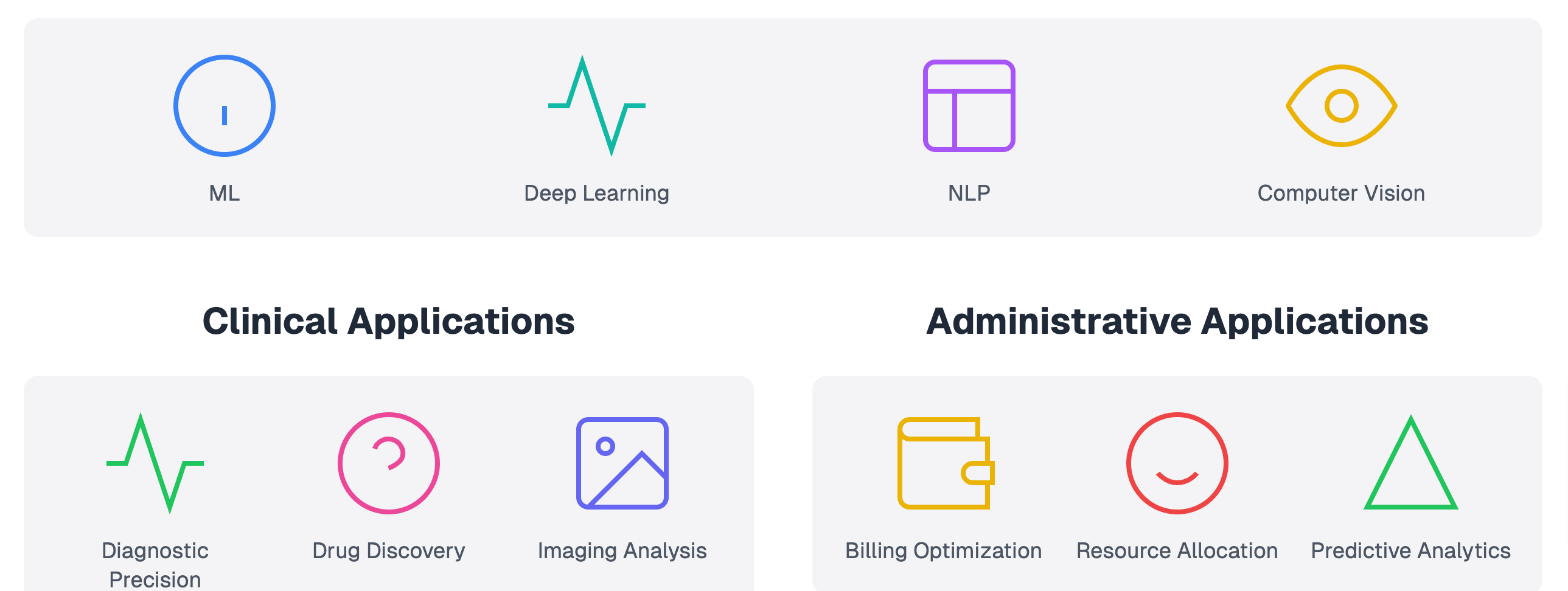
How does AI address challenges across the healthcare workflow, from scheduling to post-treatment? AI’s role in improving efficiency and outcomes
Artificial Intelligence (AI) holds significant potential to address various challenges faced by healthcare businesses at every stage of the healthcare operations workflow. Here’s an overview of common issues and how AI can offer solutions:
Patient Scheduling and Registration
Challenge: Traditional healthcare appointment scheduling is often manual, leading to double bookings, no-shows, and long waiting times for patients. These inefficiencies result in poor resource management and a subpar patient experience.
Solution: AI-driven scheduling systems use historical data to optimize appointment slots by analyzing patient arrival patterns, appointment durations, and healthcare provider availability. AI can predict no-shows and overbookings, ensuring better utilization of appointment slots, reducing wait times, and improving resource efficiency.
Check-in Process
Challenge: Manual patient check-in processes can lead to errors, such as incorrect patient details or delayed data entry, slowing the patient intake process and affecting medical record accuracy.
Solution: AI-enabled chatbots and automated check-in systems streamline the process by guiding patients through prompts to collect necessary information. These systems reduce errors by verifying data in real time and integrating with electronic health records (EHRs), making the check-in process faster and more accurate, thereby enhancing the patient experience.
Clinical Assessment
Challenge: Variability in clinical decision-making among healthcare providers can lead to inconsistencies in patient care and treatment outcomes.
Solution: AI-powered decision support systems analyze patient data, such as medical history, lab results, and symptoms, to provide evidence-based insights and recommendations. These systems help standardize clinical assessments, reducing variability and enabling more informed decisions, leading to improved care quality.
Medical Examination and Diagnosis
Challenge: Access to specialists is often limited, particularly for patients in remote or underserved areas, resulting in delayed diagnosis and treatment.
Solution: AI diagnostic tools and telemedicine platforms enable remote consultations and assist in analyzing medical images and test results. AI helps healthcare providers diagnose conditions more accurately and quickly by providing second opinions or highlighting potential issues, especially in areas with limited specialist access.
Treatment Planning
Challenge: Creating personalized and effective treatment plans involves managing numerous variables, increasing the risk of errors in the process.
Solution: AI algorithms analyze patient data, including genetic information, lifestyle factors, and medical history, alongside the latest research. This allows for the development of individualized treatment plans that are evidence-based and continuously updated based on patient progress, ensuring optimal care.
Treatment Administration
Challenge: Medication errors, such as incorrect dosages, and challenges with patient adherence to prescribed treatments, are major concerns in healthcare.
Solution: AI-powered robotic systems ensure accurate medication administration by delivering the correct dosages at the right times. AI can also send personalized reminders and educational materials to patients, improving adherence to treatment plans and reducing errors.
Monitoring and Follow-up
Challenge: Continuous patient monitoring, especially for those with chronic conditions, can be resource-intensive and difficult to sustain.
Solution: AI-enabled remote patient monitoring systems, combined with wearable technology, track real-time health metrics and use AI analytics to detect early signs of health issues. This proactive approach reduces the need for frequent in-person visits and enables timely interventions, optimizing healthcare resources.
Claims Processing
Challenge: Slow and error-prone insurance claim processing can result in denials, impacting the financial stability of healthcare providers.
Solution: AI analyzes claims data to identify patterns and predict potential issues that could lead to denials. By ensuring claims are accurate and complete before submission, AI reduces denial rates and expedites the processing, improving financial outcomes for providers.
Payment Processing
Challenge: Managing payments and account reconciliation can be time-consuming, leading to delays in revenue collection and financial discrepancies.
Solution: AI-powered chatbots and virtual assistants manage payment-related inquiries and provide immediate assistance. Predictive analytics can forecast revenue cycles and improve cash flow management, ensuring timely payment collection and reconciliation.
Medical Records Management
Challenge: Manual record-keeping is prone to errors and consumes significant time, impacting the availability and accuracy of patient data.
Solution: AI-driven EHR systems automate record-keeping, ensuring accurate, up-to-date patient information. These systems also facilitate efficient data retrieval and exchange, improving collaboration among healthcare providers and enhancing patient care.
Quality Assurance and Compliance
Challenge: Adapting to frequently updated healthcare regulations requires significant resources and time, making it difficult for organizations to stay compliant.
Solution: AI can monitor regulatory changes and automatically assess their impact on healthcare practices. AI-driven compliance tools notify healthcare providers of necessary updates and streamline the verification process, reducing the risk of penalties and improving quality control.
Discharge Planning
Challenge: Lack of post-discharge support can lead to complications and readmissions, increasing healthcare costs and negatively impacting patient outcomes.
Solution: AI-powered care coordination tools create personalized discharge plans, including follow-up appointments, medication schedules, and self-care instructions. These tools provide ongoing support through automated follow-ups, ensuring that patients have the resources for a successful recovery.
Patient Education
Challenge: Educating patients about their health conditions and treatment options is essential, but healthcare providers often have limited time and resources for effective communication.
Solution: AI-powered educational platforms deliver personalized health information tailored to each patient’s understanding and preferences. These platforms enhance patient education, improving treatment adherence and empowering patients to manage their health more effectively.
Follow-up and Aftercare
Challenge: Monitoring patient outcomes post-treatment is crucial for long-term health, but resource constraints make this difficult to sustain.
Solution: AI analytics track patient outcomes by analyzing data from follow-up visits, wearable devices, and other sources. Early detection of trends or issues allows for timely interventions, improving aftercare and long-term health outcomes.
AI applications across the healthcare operations workflow can address critical challenges by enhancing efficiency, reducing errors, supporting clinical decisions, and ultimately delivering better patient care. However, successful AI implementation in healthcare must consider ethical, legal, and privacy issues to ensure a responsible and effective transformation.
Streamlining healthcare processes using generative AI
Effective management of patient admission, treatment, and post-discharge processes is essential for maintaining continuity and delivering high-quality care in the healthcare sector. Generative AI optimizes these processes by automating tasks and personalizing care, leading to greater accuracy, efficiency, and better patient outcomes. Below are some specific processes involved in healthcare operations and the role of generative AI in this context:
Patient Admission
The patient admission process involves several steps that generative AI enhances to improve efficiency and accuracy. Initially, the system retrieves patient records, automating the extraction of relevant data for quicker processing. When a patient’s critical condition is detected, AI flags it for immediate attention, ensuring timely medical intervention. Generative AI then notifies the admission team, sending automated alerts to streamline communication.
During the medical assessment, AI analyzes data from physical examinations, tracking vital signs trends to provide real-time insights. This analysis helps healthcare providers make informed decisions on treatment plans. As part of treatment plan creation, generative AI reviews the patient’s medical history and synthesizes a personalized treatment summary, guiding healthcare professionals in making tailored decisions that best suit the patient’s needs.
Treatment Plan Management
Generative AI also plays a significant role in treatment plan management, starting with the creation of a plan. The AI system analyzes patient data to define personalized treatment goals, reviewing clinical inputs to form a comprehensive understanding of the patient’s condition. Based on historical and real-time data, the AI suggests optimal care plans that are specifically tailored to the patient’s needs.
The AI continuously monitors patient progress, tracking data and evaluating the effectiveness of the treatment by comparing current outcomes with the predefined goals. If necessary, it proposes modifications to the care plan, ensuring the patient receives the best possible treatment as their condition evolves. The AI system provides detailed summaries of patient outcomes, offering healthcare providers actionable insights to guide future decisions.
Diagnostic Testing
In diagnostic testing, generative AI assists throughout the entire process. It begins by preparing the patient for specimen collection, and generating customized instructions based on individual requirements. To ensure the safe transportation of the specimen to the lab, AI optimizes routes and conditions for faster and safer delivery.
Once the specimen reaches the lab, AI analyzes the test results, identifying patterns and correlations in the data to aid in diagnosis. It cross-verifies findings with vast historical data to ensure accuracy, automating the generation and interpretation of test reports. The AI system also cross-checks reports against historical data to verify their accuracy before finalizing the results, making diagnostic testing more efficient and reliable.
Discharge Planning
The discharge planning process is another critical area where generative AI enhances patient care. The AI system automates the generation of discharge summaries, ensuring that all necessary information is documented. It validates the discharge plan by analyzing it for any inconsistencies or missing details and suggests revisions as needed.
As part of the patient’s post-discharge care, AI customizes educational content based on the patient’s specific health needs and recommends personalized care plans. Once finalized, the AI generates detailed discharge reports and alerts the billing department automatically, streamlining the administrative processes.
After discharge, AI identifies follow-up requirements by analyzing patient data, ensuring that necessary steps are taken to facilitate a smooth transition. The system evaluates the success of the transition, generating reports and notifying healthcare providers. It automatically updates the patient’s records and sends personalized notifications to the patient about their follow-up care.
For post-discharge concerns, AI analyzes patient feedback to identify any issues. It documents all suggested actions and provides tailored solutions for each concern. Additionally, it predicts further care needs by analyzing both historical and current patient data, offering a proactive approach to managing patient health after discharge.
By integrating generative AI into these various stages of healthcare operations, the process becomes more efficient, personalized, and data-driven, ultimately improving patient outcomes and operational efficiency.
Integrate AI in Healthcare with Experts
Discover how AI can reinvent healthcare. Keen to upgrade your healthcare solutions?
How can healthcare organizations effectively implement AI to enhance patient care and operational efficiency? Strategies for successful AI adoption.
Implementing AI in healthcare requires a well-structured approach to ensure effective integration while addressing important regulatory, ethical, and technical considerations. Here’s a comprehensive guide to help healthcare organizations navigate the process:
- Define Objectives and Use Cases
Begin by clearly defining the objectives for implementing AI in healthcare. Identify specific areas where AI can bring value, such as improving diagnostics, enhancing patient care, streamlining administrative tasks, or supporting research initiatives. Prioritizing use cases like predictive analytics in diagnostics or robotic process automation in administrative workflows can help focus efforts.
- Assess Data Availability and Quality
Evaluate the availability, quality, and diversity of the healthcare data you plan to use. AI relies heavily on robust datasets for training and accuracy, so it’s critical to ensure that data is well-structured, anonymized, and compliant with healthcare data protection regulations like HIPAA or GDPR. Inconsistent or biased data can lead to inaccurate outcomes, making this step foundational for success.
- Engage Key Stakeholders
Involve essential stakeholders, including healthcare providers, administrators, IT teams, and even patients, throughout the AI implementation process. Their insights are vital for identifying challenges, ensuring buy-in, and addressing concerns. Understanding their expectations and apprehensions is key to creating a supportive environment for AI adoption.
- Select Suitable AI Technologies
Choose the right AI technologies that align with your healthcare objectives. This might involve machine learning (ML) for predictive analytics, natural language processing (NLP) for interpreting medical records, or computer vision for analyzing medical images. Evaluate whether ready-made solutions or custom AI models will best suit your organization’s needs.
- Build or Acquire AI Models
Work with data scientists, ML engineers, and healthcare experts to develop or acquire AI models tailored to your specific use cases. These models must be accurate, reliable, and interpretable to ensure they meet clinical standards and can be trusted in real-world applications. The development process should also consider how AI models will integrate with existing workflows.
- Ensure Interoperability
AI systems must integrate seamlessly with existing healthcare IT infrastructure, such as electronic health records (EHRs) and clinical systems. Interoperability is critical for facilitating smooth data exchange and enabling AI tools to support healthcare providers in real-time.
- Address Regulatory Compliance
Compliance with healthcare regulations, such as HIPAA in the U.S. or equivalent laws elsewhere, is essential when implementing AI. Security measures must be in place to protect sensitive patient data, and your AI systems should meet all regulatory requirements to avoid potential legal or operational challenges.
- Implement Ethical Considerations
Address ethical issues such as bias, transparency, accountability, and patient consent. Establish guidelines to ensure that AI tools are used responsibly, do not exacerbate healthcare disparities, and maintain patient trust. Transparency in how AI algorithms operate and deliver outcomes is critical for ethical AI use in healthcare.
- Train Healthcare Professionals
Provide comprehensive training for healthcare professionals who will interact with AI systems. It’s crucial that they understand how the AI tools work, their limitations, and how to interpret and apply AI-driven insights in clinical practice to avoid over-reliance or misuse of AI outputs.
- Pilot Projects
Start with small-scale pilot projects to test AI applications in real-world healthcare settings. Gather feedback from users, assess the effectiveness of the AI solution, and make any necessary refinements before full-scale deployment. Pilot testing helps identify challenges early and ensures that AI implementation aligns with user needs.
- Scale Gradually
Following successful pilot projects, scale AI implementations gradually. Monitor performance, address emerging issues promptly, and continuously improve systems based on user feedback. This iterative approach allows for smooth integration and minimizes disruptions to existing workflows.
- Monitor and Evaluate
Establish robust monitoring mechanisms to evaluate the performance and impact of AI systems on patient care, efficiency, and outcomes. Regular assessments help ensure the technology is meeting its intended objectives and provide opportunities for ongoing adjustments.
- Collaborate with Industry Partners
Work with AI technology providers, research institutions, and other healthcare organizations to share insights and best practices. Collaboration can accelerate AI adoption, drive innovation, and improve the effectiveness of AI solutions in healthcare.
- Stay Informed About Advances
AI and healthcare technology are constantly evolving. Stay updated on the latest advancements in AI, such as new algorithms or tools, and ensure your systems are regularly upgraded to remain relevant and effective.
- Encourage Continuous Improvement
Foster a culture of continuous improvement by collecting feedback from users, patients, and stakeholders. Use this feedback to enhance AI applications and healthcare processes, ensuring the technology remains aligned with evolving healthcare needs.
Successfully implementing AI in healthcare requires collaboration, careful planning, and a commitment to addressing the unique challenges of the sector. By following these steps, healthcare organizations can leverage AI to enhance patient care, optimize operations, and drive innovation.
Looking for a reliable tech partner? Onegen.ai offers specialized AI consulting and development services to bring your healthcare business to the digital forefront. With expertise in deploying AI solutions—from diagnostics to predictive analytics—Onegen AI empowers healthcare organizations to harness the transformative power of AI and stay ahead in the rapidly evolving healthcare landscape.
Onegen AI Development Services for Healthcare
At Onegen.ai, we develop customized AI solutions tailored to the unique needs of healthcare providers. Our strategic AI/ML consulting empowers healthcare organizations to leverage AI for enhanced clinical decision-making, improved patient engagement, and optimized treatment strategies.
Our expertise in developing Proof of Concepts (PoCs) and Minimum Viable Products (MVPs) allows healthcare institutions to assess the potential impact of AI tools in real clinical scenarios, ensuring solutions are effective and suited to the healthcare sector’s specific requirements.\
Onegen’s generative AI capabilities transform routine tasks such as medical report generation, patient data management, administrative workflows, and medical documentation. Automating these processes frees healthcare professionals to focus more on patient care.
By fine-tuning large language models to accommodate medical terminology and patient interactions, Onegen enhances the accuracy and relevance of AI-driven communications and clinical analyses.
We ensure seamless integration of these AI systems with existing healthcare IT infrastructures, such as hospital management systems (HMS), electronic health records (EHRs), and clinical decision support (CDS) software. This improves operational efficiency, supports critical functions such as predictive diagnostics, personalized treatment planning, and proactive patient monitoring, and enhances overall decision-making in healthcare organizations.
Our AI Solutions Development Expertise
AI solutions development at Onegen involves creating systems that improve clinical decision-making, automate routine tasks, and personalize patient care. These solutions integrate essential components like data aggregation technologies, which compile and analyze medical information from various sources, creating a strong foundation for predictive analytics. This capability enables the forecasting of patient outcomes and disease trends to inform strategic decisions. Additionally, machine learning algorithms are employed to tailor treatment plans to individual patient profiles, ensuring that each patient’s unique health needs are considered. Onegen’s solutions span areas like diagnostics, treatment planning, patient monitoring, and administrative workflows, ultimately optimizing patient outcomes, improving efficiency, and elevating the quality of care.
AI Agent/Copilot Development for Healthcare
Onegen develops custom AI agents and copilots that enhance various healthcare operations, enabling organizations to improve diagnostics, personalize treatments, streamline processes, and enhance decision-making. Here’s how they help:
Administrative Tasks:
- Analyze patient data, doctor availability, and preferences to efficiently schedule appointments.
- Automate claim verification, coding, and submission to reduce errors and expedite reimbursements.
- Organize and extract information from medical records, allowing faster retrieval for doctors and eliminating manual data entry.
Medical Diagnosis and Treatment:
- Analyze medical images like X-rays, MRIs, and CT scans to detect abnormalities and support diagnosis.
- Identify early signs of diseases by analyzing patient data, enabling early intervention.
- Propose personalized treatment plans based on patient data and protocols.
Patient Care and Monitoring:
- Provide answers to patient queries, offer basic medical advice, and remind patients about appointments.
- Personalize treatment plans, medication schedules, and lifestyle recommendations by analyzing patient-specific data.
Drug Discovery and Development:
- Analyze large biological datasets to identify new drug targets, accelerating the drug discovery process.
- Optimize the design of clinical trials by selecting suitable participants and reducing trial costs and timelines.
- Predict patient responses to medications, enabling more effective treatment regimens.
Research and Data Analysis:
- Analyze extensive medical datasets to identify trends, patterns, and actionable insights.
- Automate literature reviews, data analysis, and experimental design to accelerate research.
- Extract critical information from research papers and clinical trial reports, simplifying access to relevant data.
- Automate the summarization of research literature, ensuring researchers stay up-to-date on the latest findings.
In healthcare, Onegen’s AI agents and copilots greatly improve operational efficiency and significantly enhance patient care and decision-making. By integrating advanced AI solutions into healthcare infrastructure, providers gain a competitive edge, empowering them to navigate the complex medical landscape with innovative tools. These AI agents personalize patient interactions, improving satisfaction and treatment adherence. Leveraging these AI-driven tools, healthcare organizations can offer cutting-edge, personalized care, make data-informed decisions, and adapt to rapid changes, ultimately leading to better patient outcomes and improved institutional reputation in the ever-evolving healthcare market.
How AI Benefits the Healthcare Industry
Artificial intelligence (AI) offers numerous transformative benefits to the healthcare industry, enhancing everything from diagnostics to supply chain management. Here are some of the key ways AI is revolutionizing healthcare:
Improved Diagnosis and Treatment
AI has the ability to process and analyze vast amounts of patient data, helping healthcare professionals make more accurate diagnoses and prescribe the most effective treatments. By utilizing machine learning algorithms, AI can detect patterns in patient data that may go unnoticed by humans. For instance, AI can analyze medical images, genetic information, and clinical records to identify early signs of diseases such as cancer or cardiovascular conditions. This leads to earlier interventions, more personalized treatments, and significantly improved patient outcomes.
Increased Efficiency
AI can automate routine administrative tasks such as appointment scheduling, managing patient records, and processing insurance claims. Automating these tasks frees up healthcare professionals to spend more time focusing on direct patient care, enhancing the overall patient experience. Additionally, AI can optimize hospital workflows, improve resource allocation, and reduce operational inefficiencies. This results in cost savings for healthcare organizations while also improving patient outcomes through more streamlined and effective care delivery.
Faster Drug Discovery
AI accelerates the drug discovery process by analyzing large datasets to identify new drug targets and predict a drug’s potential efficacy and side effects. This reduces the time required to develop new treatments, enabling researchers to design more effective clinical trials. By rapidly sifting through complex biological data, AI can pinpoint promising drug candidates and simulate potential outcomes, allowing new therapies to reach the market in less time.
Improved Clinical Trials
AI improves clinical trials by identifying patients most likely to benefit from a particular treatment. Through the analysis of patient data and biomarkers, AI enables researchers to develop more targeted therapies that increase the likelihood of successful trial outcomes. AI also streamlines patient recruitment by identifying eligible participants more efficiently, improving trial design, and optimizing overall trial management.
Improved Patient Safety
AI-powered tools can enhance patient safety by flagging potential medical errors, such as adverse drug interactions or incorrect dosages. By continuously analyzing patient data, AI can identify safety risks and alert healthcare professionals to potential hazards before they occur. This proactive approach reduces the likelihood of medical errors, improves patient outcomes, and helps prevent harmful incidents.
Administrative Workflow Automation
Healthcare professionals spend significant time on paperwork and other administrative tasks. AI can automate many of these processes, enabling employees to focus on more value-driven activities. For example, AI-assisted documentation can help doctors and clinicians dictate notes hands-free while maintaining thorough and accurate medical records. Additionally, AI can facilitate billing, accurate coding, and seamless information sharing between departments, enhancing the overall efficiency of healthcare administration.
Virtual Nursing Assistants
AI-powered virtual nursing assistants, including chatbots and apps, provide continuous support to patients, helping with medication queries, transmitting reports to physicians, and assisting with appointment scheduling. According to studies, 64% of patients are comfortable with AI for continuous access to healthcare support. Virtual nursing assistants reduce the workload on clinical staff, allowing them to devote more time to direct patient care, where human interaction and insight are most needed.
Safer Surgeries
In surgery, AI-driven robotic systems allow for more precise operations, particularly in confined spaces, where traditional open surgeries may pose more risks. Robotic systems controlled by AI can maneuver around delicate organs and tissues, minimizing blood loss, lowering the risk of infection, and reducing post-operative discomfort. These AI-assisted surgeries often result in less scarring, shorter hospital stays, and faster patient recovery compared to conventional methods.
Fraud Prevention
AI can help detect fraudulent activity in healthcare by analyzing insurance claims for unusual or suspicious patterns. AI systems can identify practices such as billing for services that were not performed, unbundling charges, or conducting unnecessary tests to exploit reimbursements. By detecting these anomalies early, AI helps prevent fraud, saving healthcare systems millions of dollars annually and ensuring that resources are allocated more appropriately.
Optimizing Healthcare Supply Chains
AI improves the efficiency of healthcare supply chains by predicting demand for medical supplies, medications, and equipment. By analyzing historical usage patterns and current trends, AI can forecast demand more accurately, preventing shortages or overstock situations. This reduces waste, lowers costs, and ensures that critical supplies are available when and where they are needed most, improving patient care and healthcare operations.
AI is revolutionizing healthcare in multiple areas, from improving diagnostics and patient safety to optimizing supply chains and streamlining administrative processes. These advancements lead to more efficient healthcare delivery, better patient outcomes, and a more sustainable healthcare ecosystem overall.
From Automation to Personalization: The Impact of AI Agents on Healthcare Operations
AI agents are advanced autonomous entities capable of operating independently to achieve specific goals without needing constant human oversight. Unlike traditional AI models, which require human prompts for every action, AI agents are designed with a predefined objective and the ability to generate tasks and execute them based on environmental feedback. This makes them highly adaptable to dynamic environments, enabling them to process new information effectively and act accordingly.
AI agents possess a wide range of capabilities, including perceiving changes in their surroundings, influencing conditions through actions, reasoning, problem-solving, and determining outcomes. Their autonomy allows them to function without frequent intervention, while their sensory capabilities enable them to interpret complex environments. Furthermore, these agents demonstrate advanced reasoning, making informed decisions, learning from experience, and pursuing goal-oriented behavior. This adaptability and continuous improvement make AI agents essential for healthcare environments where real-time decisions and patient care are critical.
Applications of AI Agents in Healthcare
AI agents are reshaping healthcare by leveraging advanced technologies to improve patient care, streamline operations, and enhance medical research. Here are some key applications:
Medical Imaging Analysis: AI agents are employed to analyze medical images such as X-rays, MRI, and CT scans, assisting radiologists in diagnosing diseases and detecting abnormalities. These agents can precisely identify tumors, fractures, and other medical conditions, enabling earlier detection and treatment.
Disease Diagnosis and Risk Prediction: By utilizing large language models (LLMs), AI agents analyze comprehensive patient data—including medical records, genetic information, and symptoms—to help healthcare providers diagnose diseases and predict outcomes. These agents can identify patterns and correlations not easily recognized by human clinicians, resulting in more accurate diagnoses and personalized treatment plans.
Personalized Treatment Planning: AI agents tailor treatment plans based on individual patient needs, analyzing factors like genetics, medical history, and lifestyle. By offering personalized treatment options, AI agents enhance the effectiveness of therapies while minimizing potential side effects, ensuring care is more aligned with each patient’s unique profile.
Remote Patient Monitoring: AI agents monitor patients remotely, analyzing data from wearable devices and sensors. These agents can detect early signs of health deterioration or disease progression, allowing healthcare providers to intervene proactively and prevent adverse events, ultimately improving patient outcomes.
Clinical Decision Support Systems: AI agents provide real-time decision support to clinicians by analyzing patient data, medical literature, and clinical best practices. These systems help healthcare providers make informed decisions about diagnostics and treatment options, improving accuracy and reducing the likelihood of medical errors.
Healthcare Operations Optimization: AI agents optimize healthcare operations by analyzing data to improve resource allocation, reduce wait times, and enhance workflow efficiency. For example, AI can predict patient demand, optimize staff schedules, and streamline administrative tasks, leading to cost savings and improved patient experiences.
Improved Patient Outcomes: The integration of AI agents into healthcare systems ultimately aims to enhance patient outcomes. By facilitating quicker and more accurate diagnoses, providing personalized treatment plans, and optimizing operational processes, AI agents contribute to better patient care and overall health outcomes.
AI agents are transforming healthcare by improving diagnosis accuracy, personalizing treatment plans, and optimizing operational efficiency. Their ability to automate complex tasks, analyze large volumes of data, and learn from feedback makes them a powerful tool for modern healthcare organizations.
By integrating AI agents into various healthcare operations, providers can deliver more personalized care, enhance decision-making, and streamline workflows, leading to improved patient outcomes and more efficient healthcare systems.
Integrate AI in Healthcare with Experts
Discover how AI can reinvent healthcare. Keen to upgrade your healthcare solutions?
AI Technologies Used in Healthcare
AI has significantly advanced the healthcare industry, revolutionizing diagnosis, treatment, and patient care. Here are some of the key AI technologies transforming healthcare:
Machine Learning (ML)
Machine learning (ML) plays a pivotal role in healthcare by analyzing large datasets, identifying patterns, and making predictions. ML models, including supervised, unsupervised, and reinforcement learning, are used across various healthcare applications such as disease diagnosis, patient risk assessment, and drug discovery. For instance, ML algorithms can detect early signs of diseases by analyzing patient data, improving accuracy in diagnosing conditions like cancer or cardiovascular diseases. These models are also integral in personalizing patient treatment plans based on historical data, leading to more targeted interventions.
Deep Learning
A subset of machine learning, deep learning utilizes neural networks with multiple layers to process complex data. Convolutional Neural Networks (CNNs) are commonly used for medical image analysis, enabling AI systems to detect tumors, fractures, and other abnormalities in X-rays, MRIs, and CT scans. Recurrent Neural Networks (RNNs) are employed for analyzing time-series data, such as monitoring patient health metrics from wearable devices or electronic health records. Deep learning’s ability to process vast amounts of data makes it invaluable for real-time patient monitoring and early detection of diseases.
Natural Language Processing (NLP)
Natural Language Processing (NLP) enables computers to interpret, understand, and generate human language. In healthcare, NLP is particularly useful for extracting valuable insights from unstructured data, such as clinical notes, research papers, and patient records. This technology assists in decision-making by summarizing key information, identifying relevant medical literature, and supporting research efforts. Additionally, NLP can be used in chatbot applications, where it interacts with patients by answering questions and guiding them through medical information.
Computer Vision
Computer vision technology analyzes visual information derived from medical images, such as X-rays, CT scans, and MRIs. AI algorithms leverage computer vision to detect anomalies, such as tumors or fractures, assisting radiologists in accurately diagnosing medical conditions. Beyond diagnosis, these systems can also predict disease progression and treatment outcomes by identifying subtle patterns in the images. This technology enhances diagnostic accuracy, leading to earlier interventions and better patient outcomes.
Image Recognition
Image recognition in healthcare involves the use of AI and machine learning algorithms to analyze medical images, identifying patterns, anomalies, or disease indicators. This technology supports medical professionals in making more accurate and timely diagnoses. AI-powered image recognition tools have proven particularly useful in areas like oncology, where they help identify cancerous tissues in early stages, enabling effective treatment planning and improving survival rates.
Speech Recognition
Speech recognition technology is becoming increasingly valuable in healthcare, allowing clinicians to convert spoken language into written text. This is especially useful for documenting patient information, medical histories, and treatment plans. Rather than typing notes manually, healthcare professionals can dictate their observations, diagnoses, and recommendations, which are then transcribed automatically into electronic health records (EHRs). This technology not only saves time but also improves accuracy and streamlines administrative tasks, allowing clinicians to focus more on patient care.
These AI technologies are reshaping healthcare by enhancing diagnostic accuracy, optimizing workflows, and improving patient care. By leveraging machine learning, deep learning, NLP, computer vision, image recognition, and speech recognition, healthcare providers can offer more personalized, efficient, and data-driven care.
Ethical Considerations and Challenges in the Integration of AI into Healthcare
The integration of artificial intelligence (AI) into healthcare holds significant promise for improving patient outcomes, optimizing system efficiency, and unlocking insights from vast amounts of medical data. However, this integration also introduces a host of ethical challenges and considerations that must be carefully navigated to ensure that AI benefits all stakeholders equitably and responsibly. Below are some of the key ethical concerns surrounding the use of AI in healthcare:
Patient Consent and Privacy
One of the most pressing ethical challenges in AI integration is ensuring patient consent and protecting privacy. AI systems require access to vast amounts of personal health information (PHI) to train algorithms and make informed decisions. Ensuring that patients fully understand how their data will be used and obtaining their informed consent is crucial. However, explaining complex AI processes in a way that patients can comprehend remains a challenge. Additionally, safeguarding patient data from breaches or unauthorized access is critical, as the use of sensitive health information comes with a high risk of privacy violations.
Equity and Bias
AI algorithms are only as good as the data on which they are trained, and if the data used to develop these algorithms is biased or unrepresentative, the resulting systems may perpetuate or worsen existing healthcare disparities. For instance, AI tools trained on data from specific demographic groups may fail to provide accurate diagnoses or treatment recommendations for underrepresented populations, leading to unequal healthcare outcomes. Ensuring that AI models are trained on diverse, representative datasets is a significant ethical challenge that requires ongoing attention to avoid reinforcing bias in healthcare delivery.
Accountability and Transparency
The “black box” nature of many AI algorithms—where the decision-making process is not fully understandable even to experts—poses challenges in ensuring accountability and transparency. When AI systems assist in clinical decision-making, it can be difficult to determine who is accountable for errors or unintended outcomes: the healthcare provider, the AI developer, or the system itself. Patients and clinicians need to trust AI-driven decisions, and this trust is built on transparency. Ensuring that AI algorithms are explainable, and making clear who is responsible for AI-assisted decisions, is essential for fostering confidence in AI tools.
Autonomy and the Human Touch
AI’s growing role in healthcare raises concerns about the potential erosion of patient autonomy and the human aspect of care. While AI can assist in diagnosis and treatment, it is essential that it does not replace the patient-physician relationship, which is foundational to healthcare. Patients need to feel that their care is personalized, and healthcare providers must maintain the human touch that fosters trust and empathy. Ensuring that AI complements rather than diminishes patient autonomy and the human element in healthcare is an important ethical consideration.
Ensuring Ethical Integration of AI in Healthcare
The integration of AI into healthcare has the potential to revolutionize the industry by improving efficiency, enhancing patient care, and enabling more accurate diagnoses and treatments. However, ethical considerations such as patient consent, data privacy, equity, bias, transparency, accountability, and the preservation of the human touch must be carefully managed. Navigating these challenges requires continuous ethical scrutiny, the development of robust governance frameworks, and ongoing collaboration between healthcare providers, AI developers, and policymakers to ensure that AI is used in a way that aligns with societal values and healthcare principles.
As AI in healthcare continues to evolve, it is essential that these ethical challenges are addressed proactively. By prioritizing patient rights, ensuring equitable access, and maintaining transparency and accountability, the healthcare industry can leverage AI technologies responsibly to benefit patients and improve healthcare delivery overall.
The future of AI in Healthcare
As technology continues to advance, the role of artificial intelligence (AI) in healthcare is rapidly expanding. AI offers the potential to revolutionize the healthcare sector by improving patient care, lowering costs, and enhancing operational efficiency. In the coming decade, the impact of AI in healthcare is expected to grow significantly, particularly in diagnostics, treatment personalization, and drug development.
One of the most promising areas for AI growth is diagnostics. AI-powered systems have the ability to quickly and accurately analyze vast amounts of data, leading to more precise diagnoses and highly personalized treatment plans. By sifting through complex medical data, AI can assist healthcare professionals in identifying conditions earlier, improving patient outcomes. Additionally, AI systems can monitor patients’ health in real time, predicting potential health issues before they escalate into more serious complications. This proactive approach can reduce the likelihood of costly medical emergencies and help prevent chronic conditions from worsening, ultimately driving down healthcare costs.
Another exciting application of AI in healthcare is drug discovery and development. AI algorithms can process massive datasets to identify promising targets for new treatments, streamlining the drug discovery process and making it more cost-effective. The ability of AI to accelerate research means that new therapies can reach patients faster, improving access to life-saving medications. Furthermore, AI can assist in identifying which patients are most likely to benefit from specific treatments, enabling more personalized approaches to care that cater to individual patient needs.
AI is also expected to have a major impact on surgical procedures in the near future. AI-powered systems are capable of providing real-time feedback during surgery, improving precision and reducing the risk of complications. For instance, AI can analyze imaging and patient data during surgery to assist surgeons in making more accurate decisions, ultimately leading to safer, more efficient procedures. As AI continues to integrate into operating rooms, we can expect an increase in minimally invasive surgeries with faster recovery times and better outcomes for patients.
Conclusion
The integration of AI into healthcare marks a pivotal shift that will reshape medical practices and improve patient outcomes. AI’s ability to analyze vast amounts of data allows healthcare professionals to make more timely and accurate diagnoses, personalize treatment plans, and manage diseases more efficiently. Beyond assisting healthcare providers, AI empowers patients through early disease detection, personalized care strategies, and improved treatment outcomes, creating a more proactive and patient-centered approach to healthcare.
As AI continues to evolve, collaboration between healthcare providers and AI development companies will be crucial to ensuring that AI is implemented ethically and responsibly. Key challenges such as data privacy, security, and the ethical use of AI in healthcare must be addressed to maintain trust and ensure equitable access to AI-powered solutions. By developing tools that address critical healthcare challenges—such as disease prediction, drug development, telemedicine, and operational efficiency—AI will continue to transform the industry, leading to improved care quality, reduced costs, and better health outcomes.
The future of healthcare is poised for tremendous innovation through AI, making it essential for stakeholders to work together to fully harness its potential in an ethical and responsible manner. AI is set to create a more efficient, effective, and patient-centered healthcare system that will benefit both providers and patients alike, transforming how care is delivered and managed.
Engineering leaders from
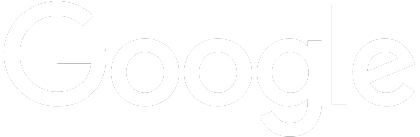
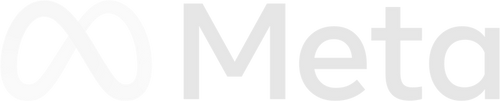
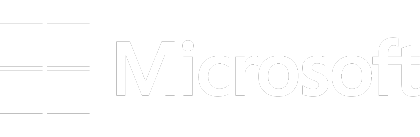
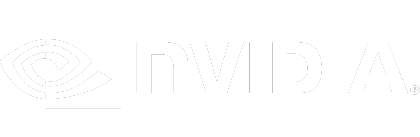
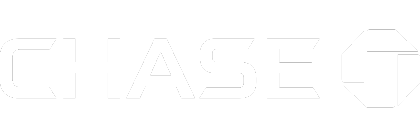
San Jose, CA - New York, NY